Generative AI is rapidly transitioning from hype to a powerful, value-driving tool across real business applications. From automating technical documentation to assisting with code generation and incident analysis, its potential is vast. However, the successful implementation of generative AI requires more than technical know-how. It demands the right strategy, use cases, and alignment with business goals. With the right AI solution, businesses can transform operations, reducing overhead while boosting creativity and agility.
In this blog post, we’ll explore the key benefits of generative AI, a step-by-step implementation framework, and practical tips to ensure a seamless adoption tailored to your IT infrastructure and strategic goals.
Importance of generative AI for businesses
Implementation of generative AI helps businesses streamline workflow by automative
repetitive task and take strategic decisions using data driven insights. The following points
highlight the importance of generative AI for businesses.
Increased productivity and efficiency
Generative AI can automate repetitive tasks like data analysis, coding, and email marketing, and generate outputs much faster than humans, freeing up employees to focus on more strategic work, enhancing productivity and efficiency.
Enhanced decision making
Generative AI can analyze large sets of data to identify patterns and generate forecasts and provide insights to make data driven and informed business decisions. It also simulates different outcomes, helping businesses assess risks, explore various scenarios, and make more informed decisions.
Hyper-personalization
Generative AI can help businesses create highly targeted marketing campaigns by personalizing content and experiences for customers, leading to increased engagement and satisfaction.
Improved customer service
Generative AI can provide 24/7 customer support based on individual needs and preferences, ensuring that customers receive timely assistance even during off-peak hours.
Increased security
Generative AI can help businesses analyze massive volumes of data and identify anomalies and potential security threats in real time, thus proactively minimizing risk and safeguarding assets.
Accelerated product design
Generative AI can provide product design alternatives and stimulate user experience, enabling the production team to visualize and fine-tune design ideas quickly. This helps businesses to significantly reduce product development cycles and encourage creative exploration.
Step-by-step guide for generative AI implementation
Now that we’ve explored why generative AI matters for modern businesses, let’s
understand the step-by-step implementation approach.
Step 1. Define your business goals
Identify the areas where generative AI can create the most impact. Look for the “why” while defining your business goals.
- Do you want to automate content creation?
- Are you looking to enhance customer support?
- Are you planning to personalize user experiences?
- Do you want to improve operational efficiency?
Setting measurable goals ensures AI investment is strategically aligned with your business objectives. It also ensures all teams are aligned on strategy, objectives, implementation, and success metrics.
Example:
A manufacturing company can use generative AI to automate maintenance guides or quality standard documentation, enhancing internal efficiency.
Step 2. Evaluate your data preparedness
Assess your existing data and identify all potential sources to implement generative AI. Ensure your team and processes are prepared to adapt to new technology.
Unify and prepare data by investing in data enrichment or cleansing activities. Organize data well, make it easily accessible, and ensure that it comes from ethical sources. Without a robust data foundation, even the most innovative AI tools will perform sub optimally or deliver questionable outputs.
Pro-tip to improve data quality:
- Identify and reduce inconsistency by conducting regular data audits
- Enhance the existing database by implementing data augmentation techniques
- Implement data security protocols to enhance data integrity
Step 3. Identify the right generative AI solutions
With a market filled with AI platforms, selecting the right solution demands scrutiny.
Look for generative AI consulting services that support your business objectives, whether you require text generation, code help, design assistance, or multi-modal models.
Also, think through whether you would require a flexible API, plug-and-play SaaS software, or a commercial-grade solution. Search for features such as scalability, integrations with systems already in use, and compliance with security measures. Make sure the generative AI consulting company you select provides transparency, documentation, and continual support for more efficient implementation and risk reduction.
Example:
Use OpenAI’s API for general tasks or fine-tune a local model for domain-specific applications like legal document drafting.
Step 4. Choose the right generative AI model
Select the right generative AI model that integrates seamlessly with your platform and fits your business requirements. Here are two commonly used models. Here are two commonly used models:
- Large Language Models (LLMs): Designed for generating text, language translation, and summarizing text.
- Diffusion models: Used for image generation, data synthesis, and reversing the process to create new samples.
You’ll need to assess model size, accuracy, token limits, latency, and fine-tuning flexibility. Also consider ethical factors like content bias, interpretability, and content moderation controls. Hire an AI consultant who specializes in generative AI implementation services to guide model selection and integration.
Example:
For instance, a legal tech startup tests multiple LLMs and chooses a smaller open-source model fine-tuned on legal documents for confidentiality and domain accuracy.
Step 5. Train and develop a generative AI model
Once the right model is selected, the next step is aligning it with your organization’s data and goals. This could involve training employees on domain-specific datasets. Monitor training outcomes carefully to ensure the model is learning the desired patterns and producing accurate results.
Collaborate closely with data scientists, ML engineers, and business teams to balance model performance with business relevance. Hire a partner who specializes in AI model development and training to accelerate success and avoid common pitfalls.
Example:
A healthcare startup fine-tunes a base LLM on anonymized clinical transcripts to help generate patient summaries tailored for doctors.
Step 6. Deploy your model with the business ecosystem
Deployment is where strategy meets execution. Seamlessly integrate your generative AI model into existing workflows, apps, or customer-facing platforms and other aspects like data preprocessing, output formatting, and scalability, ensuring the model’s responses align with business objectives.
Establish clear feedback loops with users and your technical team to gather insights and drive continuous improvement. Prioritize compliance and security to protect intellectual property and customer data.
Example:
A media company integrates a generative AI chatbot into its CMS to help editors quickly generate article outlines, with human review before publishing.
Step 7. Scale and maintain
After deployment, focus on scaling and continuous optimization. Monitor usage patterns, gather feedback, and track ROI against your original business goals. Regularly retrain the model with updated data to maintain relevance and accuracy.
Follow strong governance practices as you scale with your GenAI capability, helping you remain ethical, compliant, and aligned with business objectives. A well-maintained generative AI system not only scales operations but also becomes a key pillar of digital transformation.
Example:
An e-commerce company scales its AI chatbot across regions by retraining with new data and enforcing governance for accuracy and compliance.
Tips for implementing generative AI
With a clear implementation framework in place, here are key tips to ensure your generative AI initiative delivers real business value.
1. Be comfortable with the change
Successfully integrating generative AI requires a change in culture, moreover, a shift in thinking. It demands a new set of beliefs where we let go of cynicism, fear, inflexibility, and competition so as to instead adopt the generative AI mindset, composed of curiosity, humility, adaptability, and collaboration.
Leading the AI users and the transformation is the art of cultivating AI mindset at the top of the organization to spread along the company. Therefore, you should have a culture in the organization where every member observes this and forever questions the current state (or the status quo), they also must be comfortable with change (and having failed), always need to experiment, and be willing to learn new things. It’s about a culture that is characterized by countless queries, such as, “How to give more value to customers?” or “How to build value for the world?” and last one, “How to use technology to realize that?”
2. Pay attention to your data needs
GenAI is data dependent. Hence, you should have a data plan if you don’t have one yet. Often, you will make progress, and it becomes natural to do things you wouldn’t be able to manage before. However, those skills will need occasional refreshing.
In terms of data to use when leveraging generative AI, focus on the data that is the most suitable in solving business issues and that will lead to the achievement of strategic goals. On the other hand, it’s imperative to see indent the shelf-life of that data as well. While it is interesting to know what kind of transactions or payments occurred in your business last week or a year ago, it is far more important to know what is happening in your business at this particular moment. While you are encouraged to think about AI strategy which enables you to retain and act on information you come across, it is best to do so ad as close as possible to the source.
“According to McKinsey, 40% of C-suite executives anticipate spending more on AI in the coming year.”
Moreover, you may classify internal and external data as the two primary data types so that data analysts can determine what data are more appropriate nor how to use them. Owning your data is kind of very precious, considering the fact that the data is probably the only kind of data that your organization has. While this may well be the case, the outside data (i.e. social media data) can, nevertheless, prove to be very accurate.
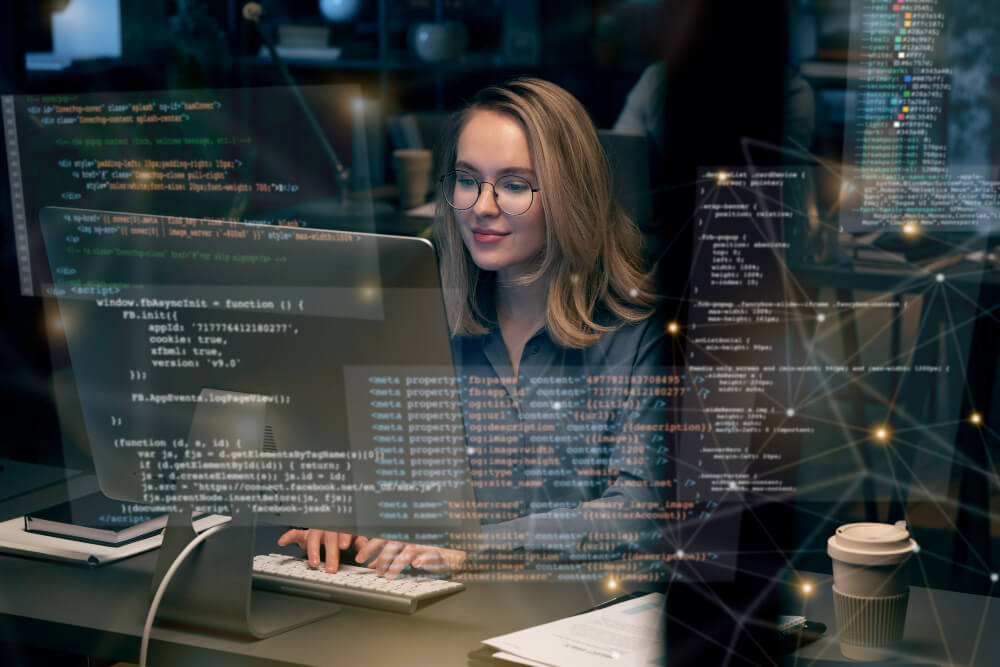
Why AI implementation projects fail and how you can avoid it
Let’s explore five reasons why organizations fail in implementing AI in business processes and how to tackle these challenges to ensure successful AI integration.
3. Generative AI is not a replacement for humans
We’ll start with the most popular myth surrounding GenAI. AI won’t do our jobs for us. We can use it effectively. The aim of applying such technology is to automate or streamline simple and routine tasks. Now, as a result of automating your mundane day-to-day operations, it gives you a chance. Generative AI cannot fully substitute the need for millions of people who are very talented and can perform creative, problem-solving and relationship-building – (but) there’s hope for improving the work for these humans.
4. Be strategic
The role of this AI application in generating business is very big. Therefore, you should check if your AI strategy remains relevant or not and if there’s any need for approval updates to use the generative AI advantages to the fullest. This translates to ending up at the point of you having to thoughtfully evaluate the possible consequences of generative AI for your current business set-up, the products or services that you offer on the market, and possibly even the overall business model that you are currently employing, and then update your entire business strategy in accordance therewith.
5. Focus on technology building blocks
There are three fundamental elements that will help you leverage generative AI successfully: first is fast, secure, and connectivity (not only at your offices but also on the go). Second is data infrastructure (i.e., the software and hardware used for collecting, storing, and accessing the data essential to your business). Third is cybersecurity, which involves using technologies to keep away malware, phishing, and other kinds of threats.
Then, the generative AI tools that straddle the foundation roost on them. Rather, you’d be using a ready-made interface such as ChatGPT or its base GPT-4 language model, having to evaluate the privacy risks involved beforehand. (For instance, put yourself in the place of whether or not you want your data to be used for training language models or even if other users will be able to see your data.)
Generative AI: Business use case
![]() |
Text generation: Generative AI performs text generation, creating individualized messages, designing websites, social networks, or email content perfectly, and customizing written text depending upon different needs. |
![]() |
Image generation: AI is turning out to be useful in fields such as design, marketing, and gaming apparently thanks to its ability to materialize images out of simple text description. It serves the task of training, architectural visualizations as well as many other things. |
![]() |
Video synthesis: The 21st century visual age needs a technology like generative AI, that fastens video production, thus helping augmented reality (AR) and advertising as well. Extensive manual editing is reduced. |
![]() |
Knowledge management: Through generative AI, it is possible to categorize and analyze large datasets, making it simpler to detect the trends and common themes used in making informed decisions and targeting strategies capable of solving a specific problem. |
![]() |
Language translation: Generative AI allows for on-the-spot language translation, the advantage of which can’t be underestimated for anyone whose position requires customer service. This will likely promote translation quality and availability further. |
![]() |
Developer experience: Generative AI performs a dual role as both an AI assistant and an information hub for developers that contribute to the development task with useful and well-structured information. |
Generative AI is still in its early stages, but its potential seems limitless. As this technology continues to evolve, we can expect to see even more innovative applications emerge across various industries. Generative AI has the potential to revolutionize the way we work, live, and interact with the world around us. It’s an exciting time to be alive and witness the possibilities that generative AI deciphers. Having said that, we are one of the early adopters and deliver generative AI consulting services according to your business needs. If you have something in mind, don’t hesitate to touch base with us.
Turn strategy into success with generative AI
Implementing generative AI is not just a technological upgrade – it’s a strategic move that can redefine your organization’s productivity, innovation, and customer experience. However, successful implementation goes beyond technology adoption; it requires clear business alignment, robust data foundations, and strategic execution at every stage. At Softweb Solutions, we offer custom generative AI services that are designed to seamlessly adapt to your unique business requirements and are strategically executed to deliver measurable outputs.
Contact our expert team for a personalized consulting session and discover how we can help you implement powerful, responsible, and scalable AI solutions tailored to your goals.